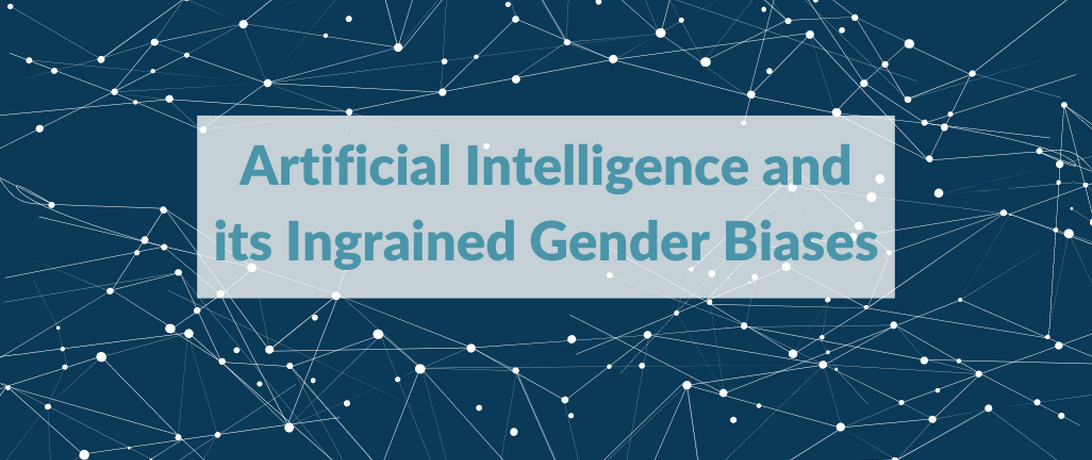
A discussion of how artificial intelligence can amplify gender biases and the need to promote the Women, Peace and Security agenda in the digital space.
The release of OpenAI’s Chat-GPT 3.5 chatbot sparked an accelerated release of similar models from companies such as Alphabet, Meta, and Snapchat. Offices are now integrating these AI tools into their software, from hiring systems to workplace support. The models’ internal perceptions of the world will skew the assistance they can provide and which groups will benefit from these new systems. Researchers are increasingly assessing and revealing that these systems display racial and gender biases, which can limit the role women will play in leadership positions. The agency that AI models are gaining brings into question how they generate their content. It is, therefore, worth exploring how these models “think” and why their internal perceptions maintain—and at times amplify—bias.
Types of AI Biases and How Large Language Models Work
In January 2023, the National Institute of Standards and Technology (NIST) released an “Artificial Intelligence Risk Management Framework” (AI RMF 1.0). The framework identifies three categories of AI bias that should be managed: (1) systemic, (2) computational and statistical, and (3) human-cognitive biases. This study considers the implications that computational and statistical and human-cognitive biases can have on perpetuating gender inequalities. The AI RFM defines computational and statistical biases as those that “can be present in AI datasets and algorithmic processes, and often stem from systematic errors due to non-representative samples.” Human-cognitive biases result from human decision-making and intentions with AI, which will affect how the AI systems train on data sets and operate once deployed. The AI RFM characterizes trustworthy AI as “valid and reliable, safe, secure and resilient, accountable and transparent, explainable and interpretable, privacy-enhanced, and fair with harmful bias managed.”
As large language models (LLMs)— deep-learning algorithms that “recognize, summarize, translate, predict, and generate content using very large datasets” — grow in dataset size and become increasingly integrated into workplace tools, their ability to generate gender bias grows. Therefore, it is relevant to consider how these models train and what data they process to learn about and understand the world. Big tech companies that have been recently releasing LLMs, such as OpenAI’s ChatGPT and Google’s Bard, have developed a “black box problem”—i.e. the LLMs’ developers are unable or unwilling to explain how the models make decisions and generate their responses. However, there are some aspects of dataset training that researchers have been able to explain. Mainly, LLMs scrape and analyze publicly available data, such as information found on Wikipedia and Reddit. Most models’ neural networks then process the text data using a “transformer,” which can read large amounts of text, identify patterns in the relations between words and phrases, and then predict which words should come next.
Recent findings also suggest that LLMs develop internal representations of the world, which allow them to reason in the abstract. For example, models can read a document and generate inferences about what the author believes or knows. Thus far, human feedback remains in the loop of LLM training through “reinforcement learning” and “iterative feedback”—when trained supervisors and users help LLMs generate better responses by flagging mistakes, ranking their answers, and setting standards for higher quality responses. The data sets and human feedback LLMs train with can reinforce computational and statistical and human-cognitive biases, leading to internalized discrimination within the systems’ reasoning.
Impact of AI Biases in the Workplace
UNESCO recently reported that women remain underrepresented in fields such as engineering and computer science. Women constitute a minority of technical and leadership roles in tech companies and make up only 12 percent of AI researchers. Therefore, female input is lacking in the training and development of the AI models at the stages when human-cognitive biases are ingrained into the models’ reasoning and internal perceptions of the world. The types of datasets that the machines are fed are also determined by a male majority, further skewing the model’s subjective worldviews to a male perspective.
Once these AI models are incorporated into the workforce, their ingrained biases become apparent. For example, in 2015, Amazon trained its automated resume screening model with resume samples of job candidates spanning a 10-year period. Their recruitment model then identified hiring patterns in the historical data where women were underrepresented. The model’s algorithm associated men with successful employment at Amazon, and discarded those resumes that were associated with women.
More recently, Bloomberg used Stability AI’s Stable Diffusion—a text to image AI generator— to test the model’s biases towards gender and racial disparities. Their team of reporters prompted the model to generate images of people associated with high-paying jobs, such as company CEOs and lawyers, and low-paying jobs, such as “fast-food workers” and “social workers.” The study found that most occupations in the generated dataset “were dominated by men, except for low-paying jobs like housekeeper and cashier.” Men with lighter skin tones were also more highly represented in high-paying jobs, including politicians, lawyers, and CEOs. Intersectional bias was therefore present in the AI’s responses. Compared to the US Bureau of Labor Statistics monthly surveys, the Stable Diffusion model significantly underrepresented gender participation in lucrative jobs and positions of power. When prompted to generate images of a “judge,” 3 percent of the images were women—compared with 34 percent in reality.
Solutions
The solutions to eliminating these biases are still being developed, but researchers are finding solutions. In March 2023, MIT researchers trained LLMs to practice logic-awareness to reduce their gender and racial biases. They trained models to “predict the relationship between two sentences, based on context and semantic meaning,” by using a dataset “with labels for text snippets detailing if a second phrase “entails,” “contradicts,” or “is neutral with respect to the first one.” The researchers found that their models became significantly less biased as a result. Using logic disassociates relationships that the machine may have found between labels such as “CEO” and “masculine” simply because of the amount of times these labels appear together. By using logic, the model dispels biases that came from using predictability. By applying similar methods and propelling more research in the field of unbiased AI, big tech companies and the U.S. government can ensure less bias in the systems that are becoming increasingly ingrained in our software.
As the examples above demonstrate, AI has not only reflected but also amplified existing gender inequalities that exist in society. This bias can lead to economic limitations and the digital exclusion of women from leadership and high-paying positions. More significant cross-sectional exclusions may result for minorities who are not well represented in the datasets used for LLMs and supervisors that train them. If AI grows ubiquitous in decision-making and hiring processes, it must be trained to promote the Women, Peace and Security agenda in the digital space. AI models that fail to reflect evolving gender equality norms will inadvertently reverse women’s progress and potentially strict female actors of the agency in creating and using AI.
Article Details
Published
Written by
Topic
Program
Content Type
Opinion & Insights